Abstract:
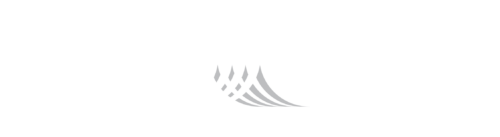
Energy Management Systems (EMS) for extensive
facilities often experience significant unplanned loads from elec-
tric vehicle (EV) operators connected to Electric Vehicle Supply
Equipment (EVSE). Facility EMS often statically curtails the
installed EVSE units to mitigate costly peak infringement. This
results in many vehicles being insufficiently charged or routinely
exceeding peak limits. The developed EVSE EMS module is
designed to reduce peak infringements while ensuring a fast and
complete charge for EV operators. This tool leverages recent
advances in machine learning (transformers, RegNet, and Deep
Q Reinforcement Learning), coupled with classical algorithms
such as Naive Bayes to learn, adapt, and anticipate impacts
of unknown charging events. This module is implemented
alongside the operating EMS at the USU Electric Vehicle
Roadway (EVR) facility. It significantly reduces costs while
ensuring efficient charging for unknown and unscheduled user
EVs.
See publication:
https://2023.ieee-itsc.org/This publication pertains to:
Systems of SystemsPublication Authors:
- James Anderson
- Mario Harper