Abstract:
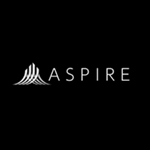
As the adoption of Electric Vehicles (EVs) continues
to increase worldwide, the efficient management of EV charging
schedules appears as a crucial challenge. This paper proposes a
two-stage optimization approach for the scheduling of EV
charging, aiming to predict vehicle departure time, allocate
maximum power while considering uncertainty, customer
satisfaction and profit of the EV charging stations. At first, the
model uses machine learning technique: Xgboost to predict EV
departure time. This is done by training the machine learning
model on unique dataset consisting of real historical data. From
the departure data, two stage optimization is solved. The first stage
is solved using the Monte Carlo Based method by solving different
scenarios and taking the average of all the scenarios to find the
expected grid power. The second stage involves real-time
optimization for power allocation, focusing on cost reduction and
a priority-based power allocation while incorporating power data
from the first stage. From the two different case studies, the station
profit was found to be $ 96.70 and $ 77.94 for 10 vehicles
respectively. Also, grid impact analysis was carried out on IEEE-34 distribution system. Overall, our research contributes practical
insights for the intelligent scheduling of EV charging, facilitating
a sustainable shift towards green transportation.
See publication:
https://www.uvu.edu/cet/i-etc/This publication pertains to:
Charging StationsPublication Authors:
- Abdullah Al Mehadi
- Orion Watson
- Abhilash Kamineni