Abstract:
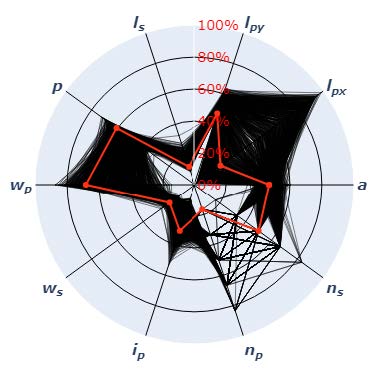
Generative Neural Networks (GNN) have demonstrated remarkable power in creating novel graphic design images from text-to-image training. This work applies GNNs to design dynamic inductive power transfer systems to make charging electrical vehicles (EVs) more convenient and cheaper. Discovering optimal and safe coil implementations (for EV and road) is challenging because of the combinatorial explosion of possible configurations, along with multiple conflicting objective functions, such as maximizing the output power, while minimizing stray magnetic fields and the volume of windings and magnetic cores. To solve the problem, a differentiable simulator and evaluator define loss functions that train a generative neural network to only produce configurations that satisfy eight given design criteria. Before training, the rate of finding successful designs is 0.005%, but within 500 training epochs, the rate becomes 98% (about 30 seconds run time). Solution diversity and quality may be further improved by applying loss functions trained on pairwise Pareto-optimal generated examples.
See publication:
https://ieee-wptce.org/program-overview/This publication pertains to:
Electrified RoadwaysPublication Authors:
- Andrew Curtis
- Md Shain Shahid Chowdhury Oni
- Abhilash Kamineni
- Regan Zane
- Nicholas Flann