Abstract:
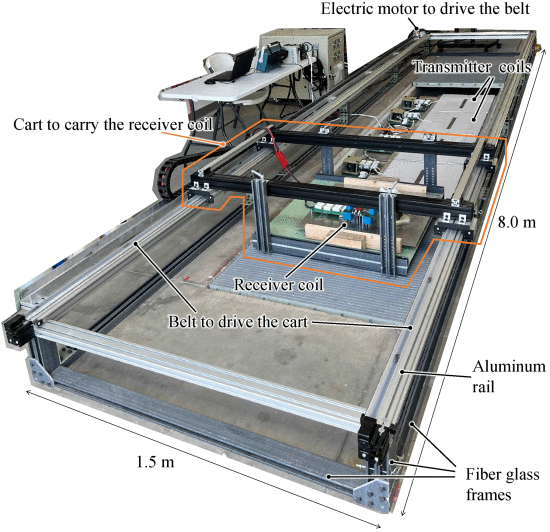
Multiple parameters with large nonlinear characteristics must be considered simultaneously to design the coil dimensions of static inductive power transfer (SIPT) systems. The design of dynamic inductive power transfer (DIPT) systems is more challenging due to the large number of parameters needed to be considered. In the conventional artificial neural network (ANN)-based design approach, optimal coil dimensions are found using ANN that has learned the nonlinear characteristics between coil dimensions and magnetic characteristics using the finite element method (FEM). However, this approach requires a large amount of training data, and it is difficult to reach an optimum design if there are many design criteria. In order to overcome these challenges, this paper proposes a design optimization method using two approaches:
improving the time efficiency of ANN training data collection by superposing the magnetic fields from the coils and improving the input value of ANN using a genetic algorithm. Design results predicted by the ANN
are compared with FEM simulation, circuit simulations, and experimental results to verify the validity of the proposed algorithm. The FEM and circuit simulation results and the ANN prediction results match with
errors of 10.2% or less for all design requirements. Experimental results are provided for a 3 kW DIPT system with four transmitter coils and an automated test rail. Comparison results between ANN predicted
values and experimental values match with an error of less than 12.7%.
See publication:
https://doi.org/10.1109/OJPEL.2022.3224422This publication pertains to:
Electrified RoadwaysPublication Authors:
- Shuntaro Inoue
- Dakota Goodrich
- Shaju Saha
- Reebal Nimri
- Abhilash Kamineni
- Nicholas S. Flann
- Regan Zane