Abstract:
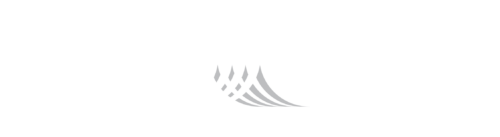
This study presents a comprehensive analysis of
electric vehicle (EV) charging scheduling at Harry Reid International Airport parking, employing a Mixed-Integer Linear Programming (MILP) based charge allocation algorithm alongside
a Reinforcement Learning (RL) approach. The primary focus is
to conduct a parallel assessment of these methodologies on the
same dataset to determine their efficacy in three critical areas:
power allocation efficiency, final state of charge (SOC) in each
vehicle, and revenue generation for the charging station. The
MILP algorithm, known for its precision in optimization under
constraints, is comparable with the adaptive and potentially
more scalable RL algorithm. This highlights the importance
of understanding the intricacies involved in employing RL for
EV charging scheduling, especially under grid constraints. Thus,
our comparison seeks to illuminate the trade-offs and potential
synergies between the MILP and RL approaches, particularly as
they relate to the increasingly complex issue of EV scheduling.
Moreover, in this study specific energy demands and logistical
nuances of the airport such as vehicle stay duration and vehicle
arrival time is considered. The result shows that, MILP had
significant customer satisfaction as well as revenue.
See publication:
https://www.kpec-ksu.org/homeThis publication pertains to:
Charging StationsPublication Authors:
- Abdullah Al Mehadi
- Orion Watson
- Abhilash Kamineni