Abstract:
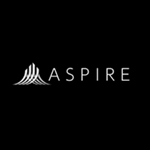
A wide variety of location and scale estimators have been developed for light-tailed distributions
such as Gaussian. Despite the ubiquitousness and importance of heavy-tailed distributions in
many business, finance, cybersecurity and other real-world applications, statistical estimation
and inference in the presence of thick tails have received less attention in the literature. We
adopt theKernelWeightedAverage (KWA) approach to location and scale estimation for samples
from heavy-tailed distributions and present a set of extensive comparisons with five prominent
competitors. Unlike nonparametric kernel density estimation, the optimally tuned bandwidth
of our KWA estimators does not necessarily converge to zero as sample size grows, except
for KWA location estimation in Cauchy samples. We also perform a large-scale Monte Carlo
simulation to search for the optimal bandwidth that minimizes the mean squared error (MSE)
of KWA location and scale estimators with simulated samples from Student’s 𝑡-distribution
with degrees of freedom 1, 2,…, 30. We further develop a data-driven approach to estimate
the degrees of freedom that best matches the observed samples using Cramér-von Mises test of
goodness-of-fit. Unlike many existing methodologies, our approach is data-driven and exhibits
excellent statistical performance. To illustrate our proposed methodology, we apply it to three
real-world financial datasets containing daily closing prices of AMC Entertainment Holdings,
Inc. (AMC), GameStop Corp. (GME) and Meta Platforms, Inc. (META) stocks to calibrate a
geometric random walk model with Student’s 𝑡 log-increments.
See publication:
--This publication pertains to:
Charging StationsPublication Authors:
- Michael Pokojovy
- S. Chen
- Andrews Anum
- J. Koomson